AI will change the affordable housing conversation for goodAI will change the affordable housing conversation for good
February 26, 2024
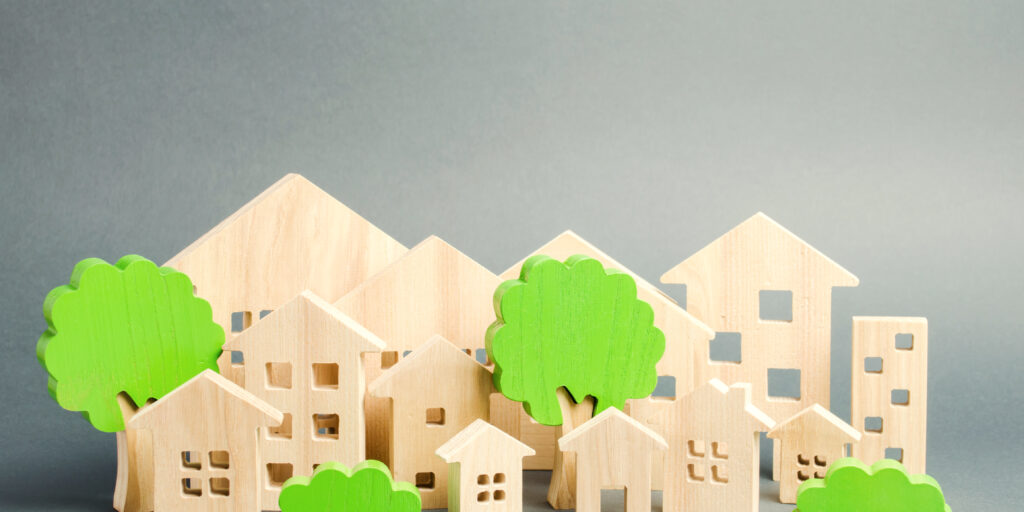
The definition of “impact” in the private housing sector is straightforward: If an activity generates more income for a property or portfolio, it’s good. If it doesn’t, it’s not.
Things are far more complex for public housing authorities. Rather than purely financial incentives, their activities are linked to societal outcomes like better affordability, lower homelessness and reduced displacement.
Outcomes like these are much harder to measure and even harder to link to specific actions or initiatives. As a result, many public housing authorities and their partners have a difficult time justifying their current activities, much less expanding their operations.
Being able to point to hard data linking their activities to social impact would help affordable housing operators and advocates garner support for new projects. Empirical evidence and data would also help local and state governments to balance costs and operate more efficiently. The sad truth, however, is that most affordable housing operators can’t make the case for themselves the same way market-rate housing operators can. They just don’t have the data.
The affordability crisis
Housing affordability is one of the defining issues of our time. Data from the real estate group Redfin indicates that only 15.5 percent of homes for sale in 2023 were affordable for a typical U.S. household. At the same time, more tenants than ever are spending more than 50 percent of their income on rent—far above the 30 percent guideline that personal finance gurus suggest.
Housing affordability has further knock on impacts for private market participants as well, such as homelessness. A recent analysis by Pew confirms that “homelessness is high in urban areas where rents are high, and homelessness rises when rents rise.” In California, where the issue has been most extensively studied, the nonpartisan California Budget & Policy Center finds that “the high cost of housing is one of the primary drivers of [the state’s] high poverty rate.”
Affordable housing, in the form of public housing projects and rent subsidies, has historically been the government’s main tool for keeping citizens housed and stable. But, as already noted, keeping people in place doesn’t earn operators any brownie points from Wall Street or from capital markets. Impact is defined in terms that are far harder to measure.
An intelligent alternative
Artificial intelligence (AI) has the potential to drive fact- and data-driven decision-making in affordable housing conversation in ways that previously have not been possible.
The private real estate sector is already using AI-powered analytics to optimize pricing, predict resident loyalty and maximize asset values. These platforms aggregate data about properties, neighborhoods, residents and trends. Armed with these data sets, leaders can use algorithms to understand pricing for new developments, acquisitions and asset optimization.
Machine learning algorithms could also be trained to measure metrics relevant to affordable housing communities, and predict what actions will maximize impact. Relevant property-level metrics might include rent affordability, housing stability, resident income and other resident demographics.
This is important because to be sustainable, cities must have sufficient housing for citizens who work in high value, but historically lower earning, sectors like education. Basically no one thinks it’s a good thing that civil servants like firefighters and law enforcement officers need to “super-commute” from hundreds or thousands of miles away to their jobs in expensive cities.
By aggregating data about properties relating to socially important metrics like income distribution and distance to jobs and training existing machine learning algorithms on these data sets, affordable housing operators could back up their claims that they help keep cities vibrant, diverse, and economically healthy with cold, hard data.
Operators could also use this data to improve existing communities. As affordable housing communities come into a preservation phase, long-term forecasting requires multiple factors around tenancy duration, repairs and costs. This is only possible with advanced analytics since a human approach will be stymied by the tools available to them.
On a larger scale, AI will reshape how government approaches housing policy. As affordable housing advocates know well, even seemingly minor changes to neighborhood can be catalysts for displacement. AI-powered simulations can predict how actions such as inserting a school in an area would impact rents and rental affordability in surrounding neighborhoods. With this information, city leadership can act to prevent disruption of communities.
Uncovering regional and national migration patterns would help cities and states plan for expected increases or decreases in population. By comparing regions where rents are rising above inflation and mean wage growth, policy makers and housing associations can be hyper-targeted in allocating policy dollars and measuring impact on rents. Together with factors like birthrate estimates, this type of analysis could inform long-term regional planning, preempting the problem of housing scarcity altogether.
What makes machine learning models so revolutionary is how they continue to improve over time. As affordable housing leaders feed these analytics engines with more data, they will soon be able to extrapolate from past data with unprecedented accuracy. This will allow governments to be more precise with how they implement affordable housing, selecting only those projects that are likely to have a significant social impact.
AI = affordability intelligence
The affordable housing conversation has long been a battleground for competing ideologies and intuitions. However, most people agree that the focus should ultimately be on ensuring that all residents, regardless of income, have access to housing. AI can provide concrete data that helps lawmakers and property owners address these concerns.
As the U.S. continues to struggle with housing affordability, affordable housing leaders owe it to the communities they serve to use all the tools at hand. AI was born to help solve complex problems like this. Let us harness it sooner rather than later so that we may better tackle this issue that affects us all.